For banks that have invested in Big Data capabilities but are struggling to generate value from them, metadata can unlock meaningful insights.
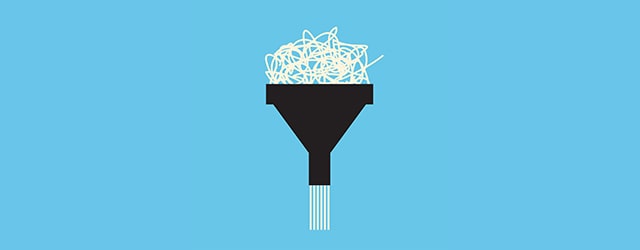
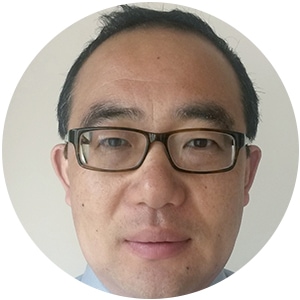
These days collecting data is easy. Figuring out what it means, however, is hard.
Banks are in possession of unprecedented volumes of data of various types, and the only way to make sense of it all is with metadata—data that describes other data, thus allowing information to be identified, discovered and stored so that analysts can unlock meaning. To put it another way: without metadata, Big Data is unmanageable.
“If you skip the metadata part, you will have problems and be overwhelmed by the size of the data,” states Zhiwei Jiang, global head of insights and data for financial services at Capgemini. “It’s one of the main reasons that banks do not derive the most useful insights out of the massive data they’ve been holding and storing.”
With customer-transaction data, consumer-location data and all the data around the Internet of Things, metadata helps streamline collection, aid integration and enable analysis of Big Data. Jiang suggests three areas of technology tooling required for metadata management.
The first is exploration (i.e., how you discover the data), and this is made possible with a good set of open-source tools along with vended ones to get data organized.
The next set of tools is for data storage, including Apache Hadoop, the open-source software framework for storing data and running applications on clusters of commodity hardware. Released in April 2006, Hadoop provides massive storage for any kind of data. With enormous processing power and the ability to handle virtually limitless concurrent tasks, it removes both storage and processing constraints. Jiang says there are lot of start-ups and advanced Hadoop vendors developing data ingestion tools to help index data in a more organized way.
By providing a consistent approach—and tools that sit on top of a wide range of differing Big Data technologies—Informatica, a data software and services company, helped Russia’s Tinkoff Bank integrate and analyze huge volumes of structured and semistructured Big Data for rapid decision-making while working with both emerging technologies and traditional data management infrastructures. “Utilizing Informatica’s Big Data Management, [Tinkoff] integrated multiple types of data on Hadoop,” says Andrew Joss, Informatica’s head of industry consulting for Europe, the Middle East and Africa. “They [thereby] improved marketing effectiveness, leading in some cases to a tenfold increase in conversion rates.”
After data discovery and data storage, the next step is analysis. “In today’s world, where all these wonderful innovations, are happening with Big Data and AI [artificial intelligence], the same three simple steps: data recovery or discovery, storage, and analytics, run to the core,” Jiang says. “In order to analyze, you need to know how to organize your offerings and work out their value and function. If you want to look, for example, to see if a customer wants a mortgage with the bank, you need the metadata around both the customer and the mortgage.”
Michael Zerbs, chief technology officer at Scotiabank, agrees there are a variety of effective and reliable tools allowing banks to leverage metadata and automate data lineage information. He says banks should establish a single enterprise business glossary and logical data model to describe each business group and its role within the organization, and each consumer domain (e.g., account customer). “Once defined, you are able to create models to describe and track the data as it evolves. Without these, automation is unreliable. The models come before the tools.”
He says Scotiabank uses many tools to support DevOps (deep collaboration between software developers and IT operations) and an agile-based environment (in “agile software development,” requirements and solutions evolve through collaboration of cross-functional teams, bottom-up rather than top-down). “Also, we are developing capabilities related to automated code development, testing, metadata management and data governance,” he says. “The data-governance tools are instrumental in providing the business with direct access to quality, privacy and data-value extraction and management controls.”
For Informatica’s Joss, banks need to go even further to ensure that intelligent data management processes cover the entire organization. “They won’t be able to generate useful insights if data from different departments remain separate—actionable information is based on companywide data. Not only that, but in order to conduct analysis quickly, it’s essential to have all your data at your fingertips whenever you need them. An agile business must ensure that its data assets are available at the drop of a hat.”
LEVERAGING LINEAGE
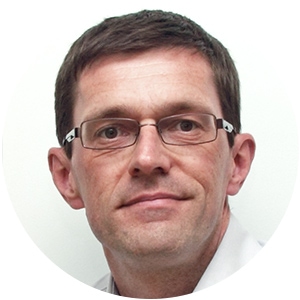
To achieve this, Joss says banks should first map out their information landscape, ensuring that their data management systems have insight into every area of the business. “Then they should consider [designing] their business [IT] around unified management policies and processes. Finally, creating a single view of the key data assets across the organization will start to unlock the value of the underlying data,” he explains. “Taken together, these capabilities will improve the trustworthiness of any insights generated.”
He also stresses the importance of clean data: “Automated management systems can help ensure that duplicates, false information and outdated entries are removed, and that the data is presented in a unified format for rapid digestion.”
Scotiabank has made great progress on a long journey to improve Big Data capability. “We continue to do more to assess, organize and report on legacy data more effectively to add business and customer value,” states Zerbs, highlighting a major shift occurring in the convergence of operational and analytical data sources. “Today, with event sourcing and data streaming, every transaction is both an event and a data point in a larger analytical dataset capable of providing real-time insight. Creating platforms that embrace this convergence rather than separate [the elements of it] is a very promising area of investment.” This real-time processing of events creates opportunities to personalize customer engagement. In future, data streaming could lead to preemptive analytics.
Among transformational initiatives relating to data, he cites the ability to meet regulatory Risk Data Aggregation and Risk Reporting (RDARR) requirements that were leveraged into a strategic business initiative to create a strong foundation for advanced analytical insights. “Data lineage is an important part of this initiative, and today it drives a number of advanced analytics use cases,” says Zerbs. “Specifically, we have developed network analytics that identify relationships and payment flows with corporate and commercial customers. And lastly, we have enabled a customer-level view of all the products, channels and transactions among all their relationships in the bank. “
Zerbs sees the ability to graph transaction sources and targets that support the “discovery’” of long paths of transactions between multiple parties that turn out to be a single entity a major benefit of these programs. “Within a single enterprise, this shows the massive power of correct identification of data as well as a practical visualization of lineage as it applies to AML [anti–money laundering], fraud, etc.”
In Europe, the General Data Protection Regulation (GDPR) comes into force in May 2018. “GDPR requires companies to give consumers greater control over how their data is stored and used, and noncompliance comes at the price of hefty fines. A comprehensive data management strategy will give businesses the insight they need to ensure that data is responsibly and securely handled, as well as the ability to provide customers with up-to-date information on request. In turn, this will help to build stronger customer relationships, ultimately driving more revenue, as well as helping to appease the regulators,” says Informatica’s Joss.
Banks lagging behind the data curve are in serious trouble—as they know they are in breach of GDPR, says Jiang. “The challenge for laggers is they don’t know where to start, and they don’t start in the right way. Then the whole process becomes inefficient, with lots of errors and overprocessing. In global finance terms, it’s the adjustment process, where producing quarterly reports makes the whole process very inefficient.”
WHERE BUSINESS MEETS TECH
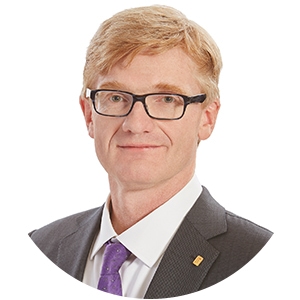
While most large banks created chief data officer (CDO) roles four or five years ago, Jiang urgently advises smaller banks to create a CDO and set up metadata functions. He also stresses the importance of looking at data for both business and technology issue: “Banks need a very comprehensive end-to-end data strategy. On one side you have the business people all wearing nice suits, and on the other are the technology people in khaki pants and T-shirts. The IT guys will think about a data source and how to mingle the mobile data with [other data] and sew them together, while the business guys are thinking about how to get the cross-sell right.”
Describing the ideal chief marketing officer (CMO) as someone who wears a suit over a T-shirt, Jiang says a data-driven CMO will work out the data sources needed for a specific outcome and then ask IT guys to merge them together. “Banks need a comprehensive data surge from both ends [business and technology],” he explains, “and to let them converge, creating a harmonious data strategy.” Zerbs agrees: “Organizations require both business and technology partners at the table to clearly define a shared vision, objectives, aspirational goals and a method to implement and measure them. They must continuously support each other and model what success looks like.”
Jiang believes the few banks making good use of data are modeling their success on big tech, e.g., Google and Apple, rather than on other banks, saying, “Google knows more about you than you know yourself—because it’s been learning all your behavior.” Like big tech, several banks have made data and AI core innovation areas. “AI will be more successful than [it has been in] previous generations,” notes Jiang, “because it will be data-driven, and the core enabler for data-driven AI is metadata.”
He does not advocate machine over human, however. “Everyone should be a data scientist—even customer reps and bank tellers,” he says. “Data is quite difficult because it is a unique asset, so you want to make sure that a lot of the leaders on the business and technology sides are also data thought-leaders—they need to know how to make journeys better and how to make money out of it.”
Banks that fail to implement strong data management will find themselves left behind. “In the connected age, data is the lifeblood of good business,” says Joss at Informatica. “Those that ignore it are putting themselves at a disadvantage—it’s essential to extract intelligence from your data assets to retain the loyalty of an increasingly demanding customer base.” And that is why metadata matters.