AI-enabled sanction screeners help ease growing headaches.
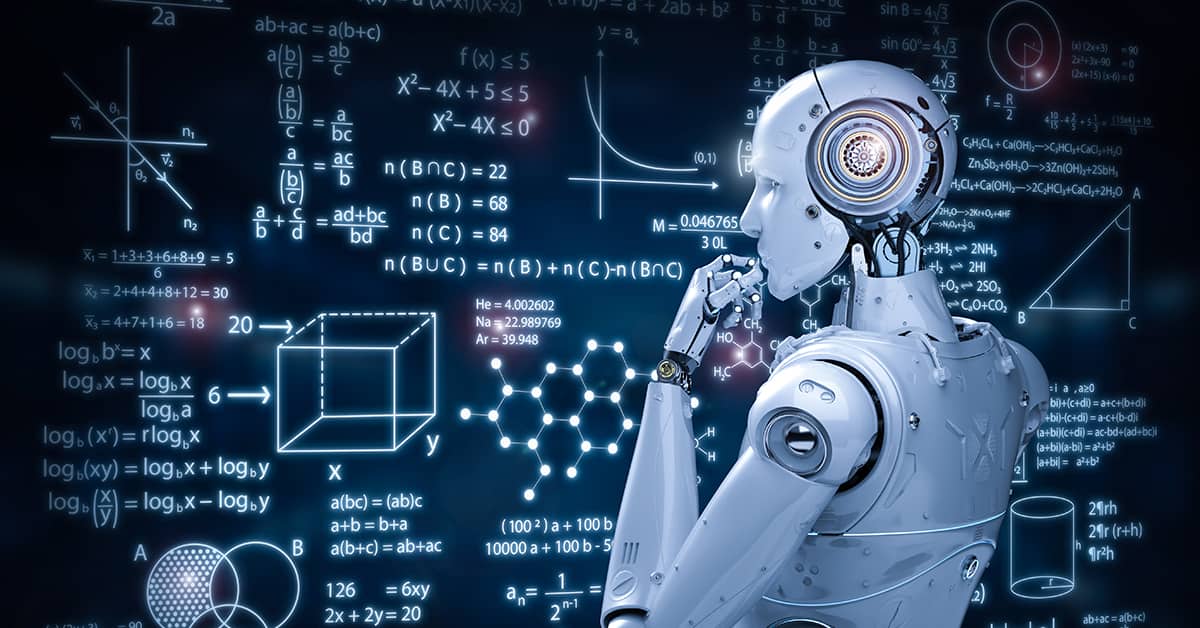
Since Russia launched its unprovoked attack on Ukraine on February 24, it has become the most sanctioned nation in history. The Group of Seven countries and other leading economies have applied sanctions on Russia’s central bank, financial institutions, sovereign wealth fund, oil and gas industry, tech sector, airlines and growing lists of lawmakers, generals, oligarchs and other individuals or entities.
As of March 9, Russia is subject to 5,581 separate sanctions, followed by Iran (3,316), Syria (2,608) and North Korea (2,077), according to Castellum.AI. Venezuela, Myanmar and Cuba escape a similar level of sanctions but still face 651, 510, and 208 sanctions, respectively.
“OFAC [Office of Foreign Asset Control] sanctions are pretty binary,” says Wren York, a strategic adviser at research firm Aite-Novarica. “There is not a lot of gray area. In fact, there is none.”
The penalties can be severe if a company or individual gets caught doing business with a person or entity on OFAC’s Specially Designated Nationals and Blocked Person List (SDN). In the case of the US Department of Treasury’s OFAC, it could fine an offender from thousands to millions of dollars and seek incarceration for up to 30 years. On the other side of the Atlantic, the UK’s Treasury approved additional penalties that its Office for Financial Sanctions Implementation can impose beyond monetary fines, due to the recent mid-March passage of the Economic Crime (Transparency and Enforcement) Act of 2022.
Since the beginning of the year, OFAC has announced six enforcement actions that incurred $11.7 million in fines, less than the 20 enforcements and $20.8 million in penalties it issued in 2021. Additionally, there has been a growing trend of OFAC issuing more fines to the corporate sector over the past few years. In 2019, 60% of the regulator’s fines were imposed upon the corporate sector. A year later, approximately 75% of the penalties went to the corporate sector, according to data from Deloitte.
Stephen or Steven?
Screening sanction lists can be time-consuming, given the number of false-positive results that analysts must investigate manually, which has led many firms to throw more bodies at the problem.
“False-positive rates are as high as 90% in the banking industry and often above that level,” says Neil Katkov, head of Risk and Compliance at industry analysis firm Celent.
Many false positives are triggered by partial matches of client data and the identified entity listed in a sanction. The percentage match that an organization can use range, depending on how comfortable it feels in responding to queries from monitors and examiners successfully.
“I’ve been to institutions where some have match rates of 80%, while others were 85% or greater,” notes Aite’s York.
Much of the manual work comes down to identifying and matching the names in question, according to Katkov. “Often true malefactors will obfuscate their names by splitting them up, putting words in the middle or leaving off a letter but in a way that the transaction will still go through.”
For example, if a sanction references a “Cindi Gonzalez,” but a firm has an account for “Cindy Gonzales,” further research is needed to determine if they are one and the same.
Screening names would be more straightforward if everyone used a standard naming convention, such as given, middle and family names. Still, some cultures include patronymic, matronymic and other elements not typically used by different cultures, which further complicate the process.
Such pattern recognition is somewhat challenging to achieve using a rules-based approach, in which someone programs a list of actions that will execute when the process encounters a specific situation.
“Let’s say there are a thousand alerts generated daily through a legacy [sanctions screening] rule engine,” explains Shaun Smith-Taylor, senior director and global head of Solutions at transaction-monitoring provider ThetaRay. “Sanction screeners will optimize those down to maybe 500 alerts. So, all they really do is save on resources.”
If third-party platform providers or internally developed systems incorporate artificial intelligence (AI) and machine learning, the process gets smoother.
Celent’s Katkov sees that taking manual results from the transaction analysts and feeding them into a machine-learning model would help cut down on false positives.
Machine learning improves efficiency, agrees Smith-Taylor, but suggests that nonsupervised machine learning, compared to supervised machine learning—where programmers check on a model’s progress and give it a nudge if necessary—can capture a wider breadth of transactions.
“The good thing about people is that they usually behave in a specific way that has a pattern that is predictive,” says Smith-Taylor. The previously unknown patterns that an unsupervised machine-learning model can identify after consuming millions of training transactions cannot be identified by a rules-based engine, since rule writers would need to be aware of a possible situation before they wrote a rule to address the new pattern, he added.
The next step in sanction screening likely will be the use of natural language processing (NLP), the branch of AI that seeks to have computers understand text and speech as people do, which would help put data into a better context, says Katkov.
“If the word ‘Moscow’ came up in a transaction, it could refer to a street name, a US town or Russia’s capital,” he explains. “Applying simple NLP could help determine if that transaction should be flagged.”
Next Steps
Whether to adopt a rules-based sanctions scanner or an AI-enabled one is a false dichotomy for organizations.
“I would say that AI and rules engines complement each other,” says Ted Sausen, an anti-money laundering (AML) subject matter expert at customer-interaction platform provider NICE Actimize. “It is more saying, ‘Here’s the output, now I’m going to put machine learning on top of it to weed out the noise.’”
“It is more about enhancing than replacing,” adds Adam McLaughlin, global head of AML Financial Crime Strategy & Marketing at NICE Actimize.
Eventually, Celent’s Katkov envisions a completely automated tagging process whose output would still be sent for review.
He doubts that future systems would be considered black boxes, since regulators still want to know how and why specific decisions were made. “There is a huge amount of work being done in AI regarding explainability,” says Katkov. “Behind even deep-learning and neural-network types of algorithms, regulators demand explainability.”
In the meantime, sanction screening capabilities are moving beyond financial institutions to other heavily regulated industries such as insurance and gaming firms, according to McLaughlin: “I’m not saying that the corporate world doesn’t need it, because it absolutely needs it.”
“We are seeing a lot of interest from corporates,” agrees Smith-Taylor. “Corporations see embedded finance as part of their product set.”