Digital transformation of business operations comes with risk.
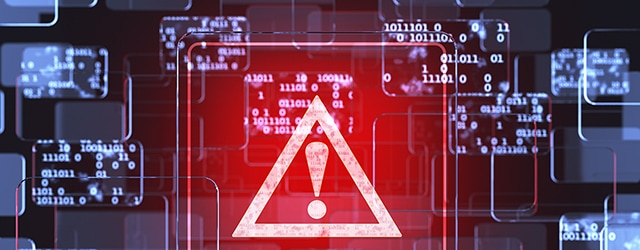
As corporate finance operations become increasingly integrated with information technology, some treasury officers are learning the hard way that procedures govern the implementation of automation just as they govern financial reporting.
Unlike financial reporting, automation is a much newer field and there is still a lot of latitude on the implementation side. Not all conceivable mistakes have been made yet, so the rulebook is still a work in progress. The technology that underpins automation is constantly changingas well, yet competitive pressure is pushing firms to implementmachine learning projects before their implications are fully understood and planned for.
Machine learning—less precisely but more popularly called artificial intelligence (AI)—allows computers to leverage acquired data toperform tasks more efficiently without explicit programming. This is how Netflix figures out what movies you want to watch. Just as systems based on machine learning sometimes guesswrongly about a person’s cinematic tastes, so too can they guess wrong about cash management projections, production fault tolerances or customers’ creditworthiness.
Research and advisory services provider Gartner lists ways this same technology can be applied to corporate finance: cash allocation, digital order entry, responding to vendor inquires to name a few.
Thepossibilities for using AI-enhanced systems or bots are endless and so are the opportunities for those systems to run off the rails.
“I’ve heard it said that ‘everyone should have a bot, almost like a pet,” says Kaush Oza, head of emerging solutions at management consultancy RGP. “But what happens if you don’t train it?”
Oza recalled one instance in which the implementation of an AI-infused financial and human resources suite nearly failed because the teams developing and implementing it couldn’t agree on how the suite should work, what it should run on or what it should actually do. He tells Global Finance that only by adding 50% to the project’s timeline and costs was the firm able to get the AI to properly align with management goals.
Recent history is replete with very public failures of AI implementationsaccording to Tech Republic, from face recognition software that got fooled by masks to accident-prone self-driving cars to chatbots that inadvertantly reflected racist or sexist prejudices of their developers. All of these fiascos have led to back-to-the-drawing-board moments and drawn greater scrutiny from management.
IT consultant and entrepreneur Joe Procopio posits that errors in implementing AI systems are more human than robotic. He starts with the example of scheduling meetings, a process that ought to be easily automated but isn’t.
“The use case for automation here is totally valid and the solution is totally valuable,” he blogs. “It is super, super easy to write the script that can offer options, take requests, even prioritize some preferences, and slap a block of time on two digital calendars. So why does this fail more often than it succeeds?”
Because people simply do notkeep their work calendars updated.
Although the digital transformation of corporate financeis still in its infancy, much can be learned and adapted from the governance of IT in general, a field that CFOs are increasingly if reluctantly learning more about. It boils down to developers, infrastructure specialists and business owners conferring and agreeing throughout the entire project timeline: from establishing a vision and configuring a solution through execution and service delivery.
At risk, according to Oza, is not just time and money. Repopulating a database or removing a logical loop is inconvenient and might even be expensive. But as the use of bots and machine learning becomes more popular, these technologies are increasinglycustomer-facing. That means that the possibility for reputational risk expands as well.