New data-crunching platform from Nasdaq mixes everything from central bank statements to celebrity Tweets to uncover actionable investment trends.
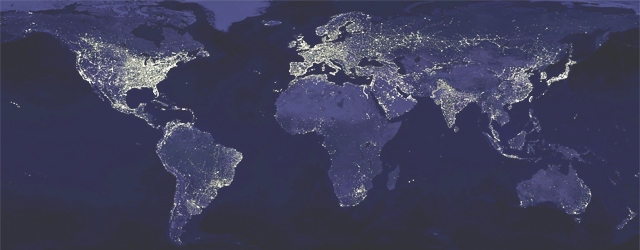
Which gossip sites and celebrity Twitter feeds do you check before making an investment? None? Maybe you should. It seems that supermodel Kendall Jenner and America’s daughter-in-chief Ivanka Trump can impact the price of a stock as much as fundamentals or expert opinions.
Kendall’s recent Pepsi ad, for example, was a fiasco on social media, but not on Wall Street: Pepsi stock surged during at the height of the scandal and dipped after the company’s apology. When president Donald Trump condemned luxury retailer Nordstrom for the decision to drop his daughter’s fashion label, Nordstrom’s stock leaped by nearly 7%. With the right analytics, you might have foreseen those outcomes and made a well-timed investment.
That’s not exactly how Nasdaq is pitching its new technology platform, Nasdaq Analytics Hub, but that is the elusive promise: It can provide customers with insight derived from applying machine learning to both structured and unstructured data—not just technical factors, but information generated analyzing social media and retail investor sentiment together with central bank communiqués and event-based signals—that will give investors a competitive edge. The goal is to create new cues to market moves that investors might otherwise miss or not be able to access.
“The confluence of massive amounts of data being produced and our customers’ desire to explore broader opportunities led to the creation of the Analytics Hub,” explains Terry Wade, senior vice president and head of business development and product for Global Information Services at Nasdaq. “There is a highly competitive landscape for the use of this data: combining unstructured and structured data through the use of a wide variety of machine intelligence methods and delivering that in a form that could be used by machines or humans is our over-arching strategy.”
Not that long ago, such large volumes of data could not be vetted efficiently. Now, new technologies have lowered the cost for effective data mining. Software can “crunch” massive amounts of information in record time, distilling valuable inputs, establishing connections and discovering new patterns: in short, giving customers a real-time competitive edge. This is particularly crucial for high frequency traders: whether it is the news of an earthquake hitting 5.000 miles away, a surge in “likes” related to a product, or a security breach at a company, knowledge before others is power. A single tweet out of the 500 millions sent every day can be the difference between making a bundle and losing your shirt.
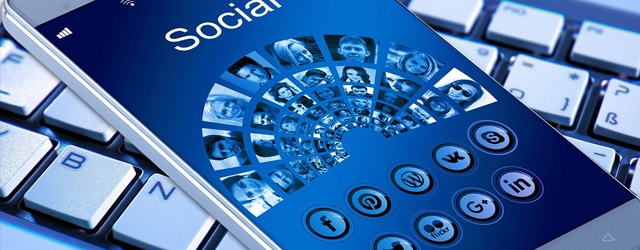
To create the platform, Nasdaq partnered with several startups, such as iSentium, which mines Twitter feeds and other social media content; PredictWallStreet, which aggregates retail investor sentiment; and Prattle, which uses language-processing software to guage the level of hawkishness or dovishness in statements from central banks. Nasdaq subsidiary Dorsey-Wright will provide indices, research and investment strategies, while Lucena Research’s machine learning-based quantitative analysis will help validate and back-test the data.“We initially scrub and normalize the partner data,” says Wade, explaining the validation process. “Then we thoroughly review the example use cases that the partner has previously created. In addition we use various machine learning methods to create value-added signals and make the information more actionable.”
Nasdaq plans on bringing more data sets to its platform, according to Wade. “What surprises me the most is that while these new data sources are fabulous individually, the real power comes when you allow the machine intelligence algorithms to find the signal from combining the data from multiple partners,” says Wade. “Over time you see the algorithms learning how the different data sets overcome the weaknesses of the others to produce better outcomes.” With 2.5 million exabytes (that’s quintillions) of data produced on the internet every day, one can’t help but wonder how fast an algorithm can really learn.