Scott Zoldi, Chief Analytics Officer of FICO, speaks to Global Finance about the role of artificial intelligence in the financial space.
Global Finance: What is driving the latest wave of excitement around artificial intelligence?
Scott Zoldi: A good number of AI applications have been in place for up to three decades, such as those addressing fraud—where we use machine learning routinely. More recently, though, generative AI has really captured the public’s attention, and it’s no longer the preserve of academics or specialists. The power of these technologies has gradually been made available to everyone.
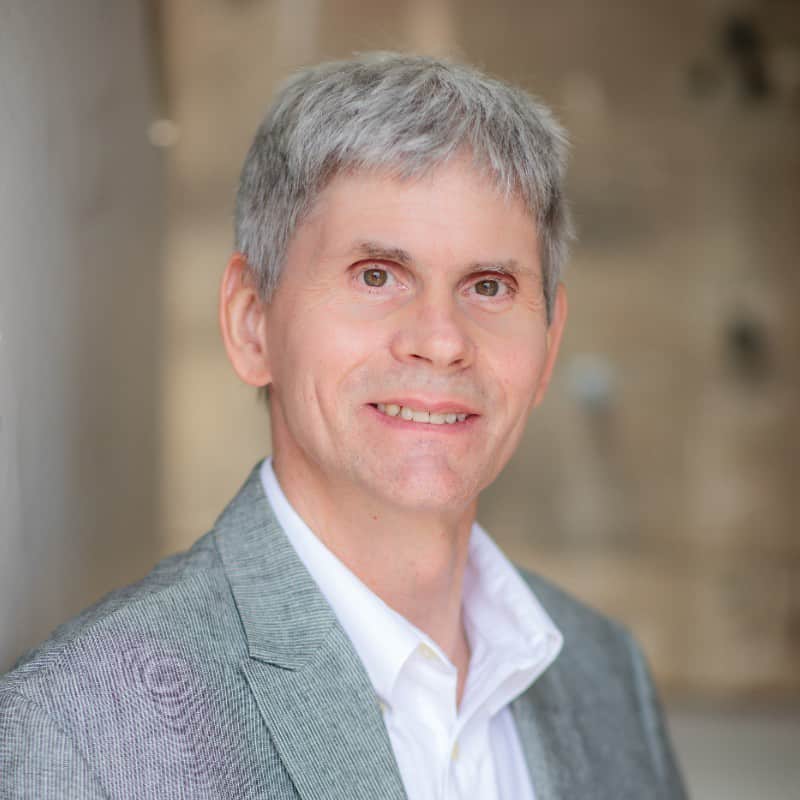
The ability of large language models to converse with us, and to impress us, has opened new opportunities for people to access new information and insight. I think this current ‘hype cycle’ is down to the fact that these tools are genuinely impressive, and interesting to play with.
The popularity of generative AI has also sparked concerns around regulation, which has started to produce an interesting amount of dialogue—whether you’re a fan of generative AI, and you’re trying to find an application that is responsible; or whether you are concerned about the potential negative impacts.
Ultimately, though, the accessibility of the technology—where even our kids can use it—is the main driver here.
GF: With the raft of regulatory activities and approaches being developed around the globe, do you foresee a danger that the resultant landscape may become over complex and burdensome?
Zoldi: There’s an ongoing debate in the US about whether regulation reduces innovation and growth and could potentially reduce the good that could come from AI. Members of the technology community, including myself, propose that innovation and regulation can coexist, however; that regulation and guidance can spawn new innovations in ways that can solve problems in a responsible AI fashion. But the debate in the US is still structured on those two forces being opposed.
That said, the Biden Administration has talked about the fact that AI, if not used properly, can create bias and systemic discrimination or bias, so we are looking very carefully at what happens in the European Union with its new AI Act.
Companies that operate globally, including FICO, must look at the totality of these regulations. I think they will all come to a common ground eventually.
One of the challenges within the regulatory sphere is that there is not a lot of specificity on how you meet explain-ability or fairness. And this is one of the things that I’ve spent a lot of my energy on recently—focusing on how organizations can demonstrate that they have met the many principles of a regulation or a guidance, or a set of best principles, because there are a lot of these and you have to choose which ones you are going to follow.
I’ve been advocating for governance standards in model development that are defined at the corporate level, to demonstrate how organizations approach, and enforce them. I think that’s one of the ways organizations can respond to developments and guidance in this ever-evolving regulatory environment.
GF: What is your view on the issues raised recently by the Center of AI Safety and some of the Big Techs about the potential societal risks of AI, as they relate to its application across financial services?
Zoldi: This is a topic that must be discussed at a global level, so I’m glad that it’s being tackled. In my view, organizations need to understand and take responsibility for the fact that they are [deploying] human-in-the-loop (HITL) machine learning development processes that are interpretable. We cannot hide behind the black box; we need to make sure that we are using transparent technologies so that we can demonstrate concretely that these models are not causing a disparate impact or discrimination towards one group versus another.
At FICO, we believe that we must demonstrate concretely that we are not creating bias with respect to the development of these models. So, I think that the current dialogue is powerful and that the outcome could be a set of acceptable machine learning technologies.
The first step is to recognize that if a machine learning model is put into production with a challenge from a bias perspective, it can propagate that bias. There are methods, such as interpretable machine learning and other technologies, that will expose that bias, and companies that follow responsible AI principles and practices will recognize that and remediate that in their analytics.
GF: How confident can businesses and consumers be of an ethical future for AI in the financial ecosystem?
Zoldi: Consumers need to be educated about the fact that AI makes mistakes. As I often say to our clients and industry colleagues: all models are wrong and some are useful. So, the first step here is an acknowledgement that all models make mistakes, and better models make less mistakes—and if we can use these better models and develop business models around that, then that’s important.
I think the other aspect of this is about getting out of the hype cycle, such as the current one around generative AI, such that we stop believing that machine learning models are always right. And I do perceive the temperature to be coming down on that now.
The next step is around transparency. So, in a similar way to how consumers provide consent to share their data for specific purpose, they should also have some knowledge of what different AI techniques a financial institution is using. Part of that is about meeting regulation, but the other part lies in acknowledging where certain algorithms are not acceptable, and that other algorithms are better.
All this will take some time but the more we have these conversations about transparent machine learning technologies, and organizations can start to demonstrate that they meet the necessary governance principles, customer confidence will improve. What is fundamental to this is ensuring that models are being built properly and safely. This is what will start to establish trust.
This is a complicated science, though, and not every organization is best equipped. Our recent survey with Corinium showed us that just 8% of organizations have even codified AI development standards, for instance. Insisting on understanding how organizations define model development standards will be amongst the things that consumers will need to know or ask—in the same way that they currently have expectations around how their data is being used and protected.
GF: Does AI pose a genuine systemic cyber threat?
Zoldi: From a cyber perspective, there’s always a risk. Adversarial AI is one such risk—where information can be injected into datasets inadvertently, and models built with that information. This is where the concepts of interpretable machine learning and looking at what that machine learning is coming up with and its relationship with what it’s learning—and then having a human being establish whether it is acceptable or palatable—is fundamentally important.
I generally say that all data is biased, dangerous, and a liability—and that’s from a model development perspective. So, when we build models, it is best to assume that the data is already dirty and dangerous. When we build the model, we must make sure that we understand what it has learned, whether we think it is a valid tool, and then apply that.
It is so important that we don’t just create a machine learning model based on a set of data that we either try to clean or don’t clean, and then just deploy it, because no one’s going to be able to clean all the issues in our data—whether it be societal bias, or a sampling bias, or all biases that we would get from a data collection perspective—let alone criminal activity—but we all have an opportunity to seek to understand what’s in the machine learning model, and choose to use technologies that are transparent.
If you think about machine learning as a tool, versus a magic box, then you have a very different mentality, which is based on needing to understand how the tool works. I think that’s how we circumvent a lot of these major risks. But for sure, cyber and data security is a big concern, because models learn from data and we cannot rely on that data being safe to use.