Viola Hechl-Schmied, product owner for machine learning at ION Treasury, lifts the lid on the AI-based tools the company integrated into its platform to help treasurers make quicker and more accurate cash forecasts.
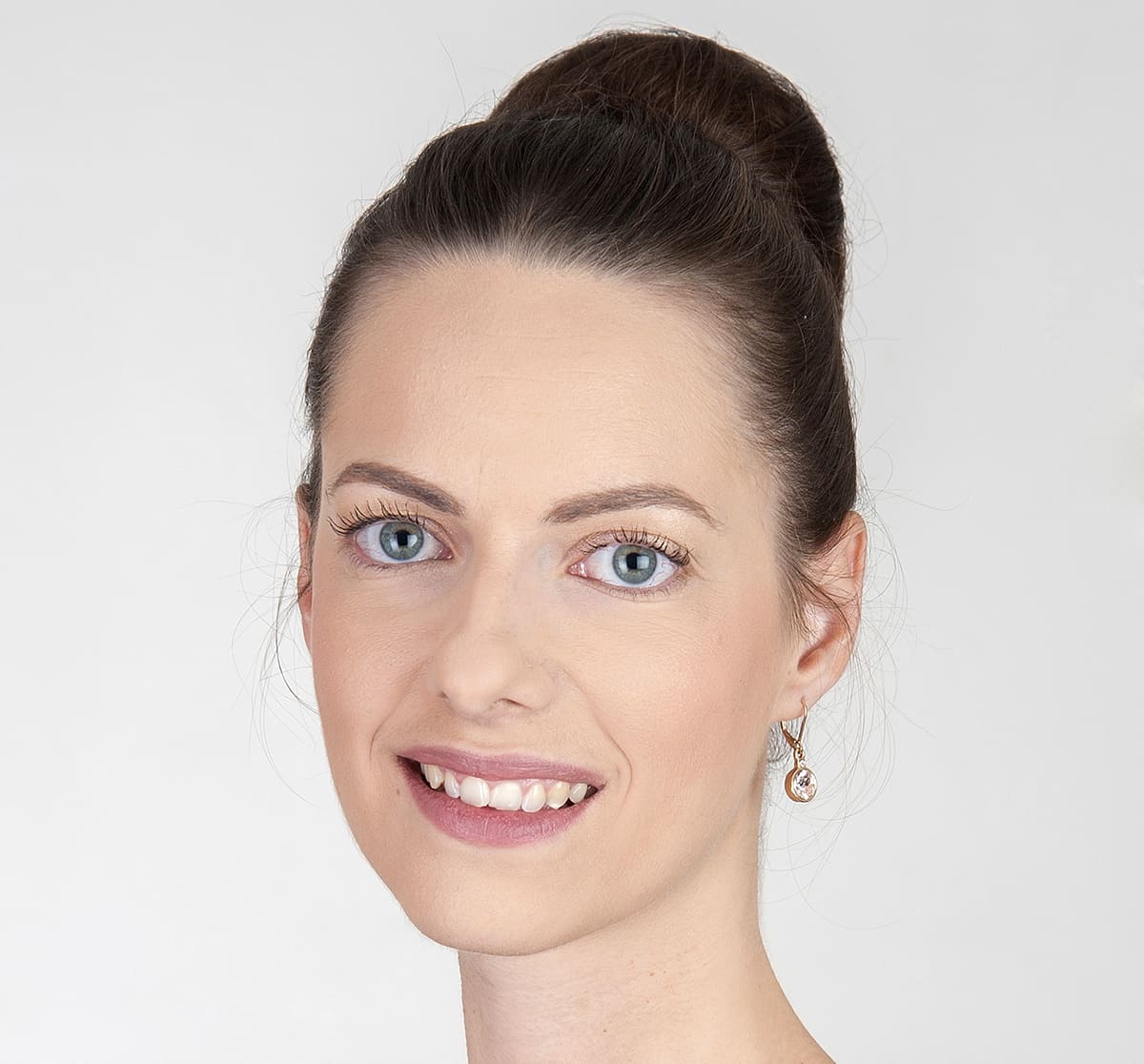
Global Finance: Which types of companies leverage ION Treasury’s machine learning and artificial intelligence (AI) for cash forecasting?
Viola Hechl-Schmied: Most companies have an existing cash forecasting process that works, but there is room for improvement. The treasury teams we work with rely on their cash forecast for planning activities over short- (six-day), medium- (13-16 week) and long-term (six-month to a year) liquidity planning. Right now, we offer the machine-learning capability for cash forecasting in three of our treasury management solutions–Reval, ITS and IT2–but we plan to integrate this functionality in all our treasury management systems.
GF: How have customers responded to ION Treasury’s new tools for cash forecasting?
Hechl-Schmied: So far, customers tell us that they like how fast it is and that the data is already available in the treasury management system. So, everything they need is all in one place. Our solution is not just take it or leave it. Users can analyze the forecast produced by the machine-learning models against actual cash flows, and run scenarios on their projections and adjust them.
Typically, liquidity planning or cash forecasting processes were manual and time consuming. We wanted to see how we could use machine learning to produce more-accurate forecasts and minimize the effort involved in storing and collecting the same data, again and again.
We want to free treasurers from repetitive tasks to focus on analyzing forecasts to improve decision-making related to their usage of cash and liquidity planning. They can enrich the data on which the machine-learning models are trained, produce forecasts for the different categories of cash they use every day and come up with a forecast six months or a year out. Usually, it would take a day to come up with a forecast for a single category of cash. Using machine learning, they can now do that in seconds.
GF: Does the treasurer need to be tech savvy or employ data scientists to effectively use the models ION Treasury provides?
Hechl-Schmied: We offer multiple machine-learning techniques. It’s not all neural networks. We also use regression models, which are easy to understand. Treasuries won’t need a data scientist. We think about how we design a neural network and how it is best suited for treasury data. We don’t just send customers pretrained models. We want to develop algorithms that can be sold to all customers and trained on their data.
GF: Is AI a silver bullet for solving companies’ cash forecasting problems?
Hechl-Schmied: AI is a journey. There is no expectation that companies will suddenly shift all their cash forecasting and apply only machine-learning techniques. However, companies need to understand their data and trust in AI. To help build that trust, we encourage treasurers to run their existing cash forecasting processes in parallel with machine learning to compare the outputs. By doing that, they can understand what is happening with the machine-learning output, compared to actuals, so they don’t need to 100% trust it blindly.
Using machine learning, I’m confident we can crack this challenge and get them to a place where the effort that goes into cash forecasting is significantly less and their decision-making is enhanced.